With maternal healthcare continuing to highlight global disparities, innovations such as BabyChecker are working to bridge the gap. Designed to provide antenatal screening in low-resource settings, BabyChecker combines artificial intelligence with affordable ultrasound technology, and years of academic research.
This solution is the result of a long-standing collaboration between Delft Imaging and the Diagnostic Image Analysis Group (DIAG) at Radboud University Medical Center (Radboudumc) in the Netherlands.
Academic excellence in AI for healthcare
Radboudumc is one of Europe’s leading academic hospitals, internationally recognized for research in medical imaging and artificial intelligence. The Diagnostic Image Analysis Group (DIAG) within Radboudumc develops AI and machine learning solutions for radiology, pathology, ophthalmology, and ultrasound.
Their work includes:
- Deep learning applications in medical imaging
- Automated image analysis for early detection
- Decision support tools for clinicians
- Global collaborations in AI-driven diagnostics for low-resource settings
DIAG has contributed to several open-access AI tools in breast cancer detection, lung disease screening, and fetal health monitoring. Their focus on open science and academic rigor continues to inform the technical foundation of BabyChecker.
From CAD4TB to BabyChecker
The academic journey that would later lead to BabyChecker began in 1997, when Professor Bram van Ginneken conducted his PhD research on the automatic detection of tuberculosis in chest radiographs. In 2008, Delft Imaging and the Diagnostic Image Analysis Group (DIAG) at Radboudumc formally began collaborating to develop CAD4TB, starting with a jointly funded research project.
CAD4TB was CE marked in 2014 and is now deployed in over 75 countries across Africa and Asia. This longstanding partnership in medical AI laid the foundation for BabyChecker, which applies similar principles of accessibility, automation, and clinical validation to maternal health.
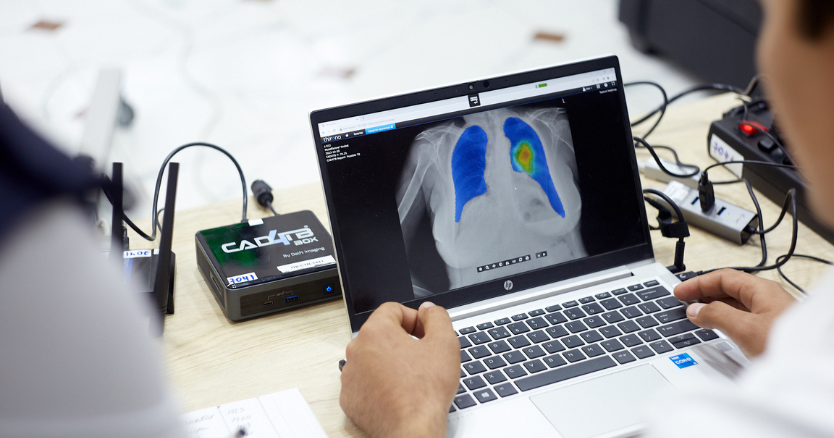
A research-driven beginning
The development of BabyChecker began as a research project between Delft Imaging and Radboudumc. What started as a PhD thesis soon evolved into a scalable solution for antenatal screening in underserved areas.
“BabyChecker was born to address the issues that impede early identification of pregnancy risks. It was envisioned as a referral tool, a screening system where risks can be identified, patients referred to a proper center for confirmation, and timely action can be taken,” said Sofia Sappia, PhD candidate at the Department of Medical Imaging, Radboudumc.
Early-stage research explored how low-cost probes could be used for standardized ultrasound protocols. A pivotal moment came in 2016, during a workshop in Ethiopia, where midwives with no prior ultrasound experience were trained to use the system in just a few days.
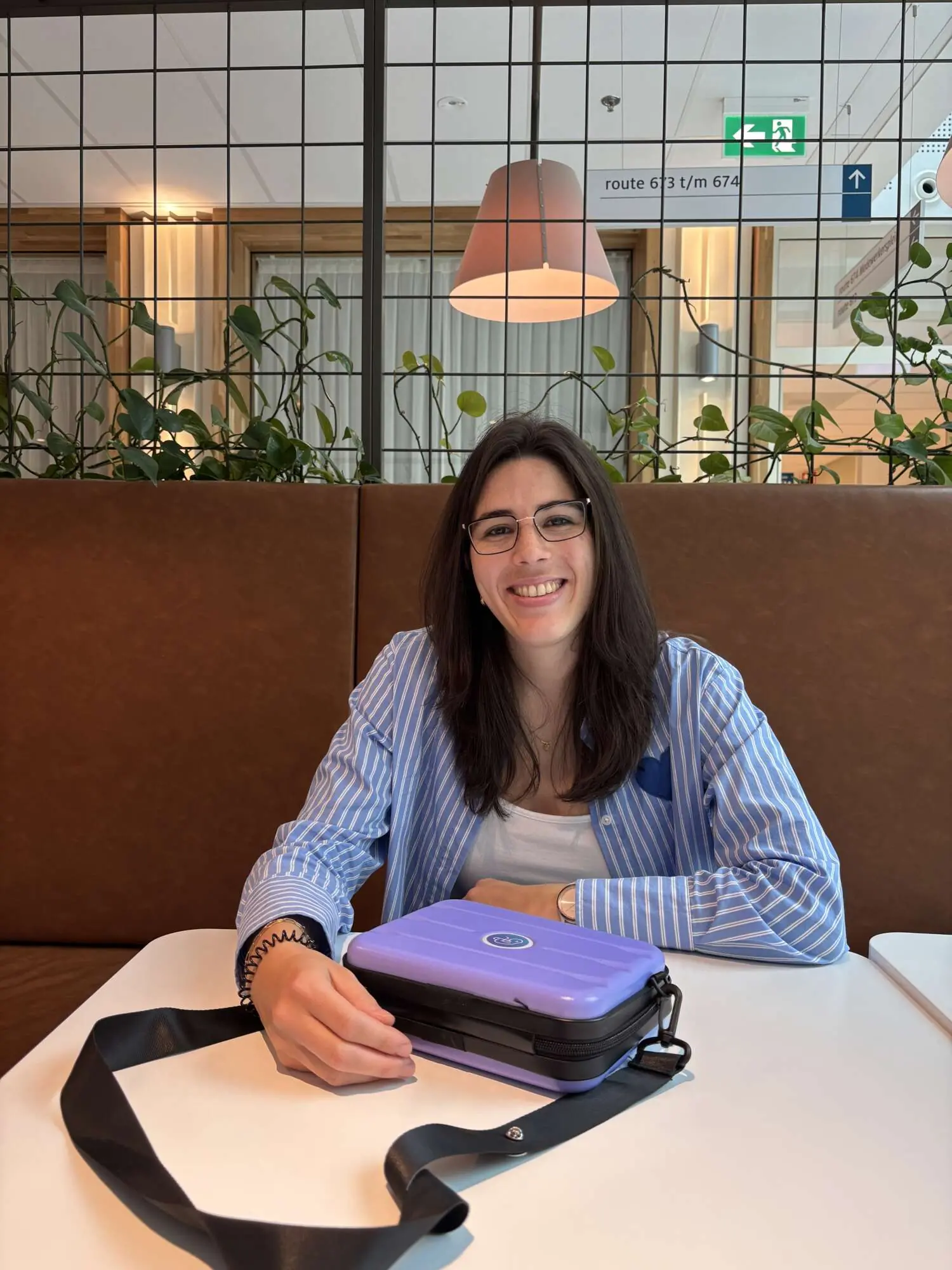
Technical approach: Standardized sweeps and AI analysis
BabyChecker does not require clinical expertise to operate. Instead of acquiring textbook-perfect ultrasound images, users follow standardized sweeps across the abdomen.
Despite using lower-cost devices with reduced image quality compared to high-end machines, research has validated that the image quality remains sufficient for the clinical measurements needed in antenatal screening.
The AI components of BabyChecker handle critical functions:
“For gestational age estimation, we identify the fetal head in the scan, segment it by labeling all corresponding pixels, and then fit an ellipse around it, similar to what sonographers do in clinical practice. This allows us to measure head circumference, which we then use with growth curves to estimate gestational age.”
The system also uses AI to determine fetal presentation, identify multiple pregnancies, and locate the placenta, generating a comprehensive report with minimal user input.
Validation in clinical and field settings
Radboudumc plays a central role in validating BabyChecker’s performance. Researchers collect data from clinical and field implementations to compare BabyChecker’s output with standard ultrasound results.
“To ensure it works reliably, we need clinical reference data – actual ground truth. That’s why we collaborate closely with the prenatal department here at the hospital, involving both sonographers and midwives who perform routine scans,” added Sofia.
This validation ensures that BabyChecker performs consistently across various healthcare settings, including outreach environments where resources are often limited.
Current research priorities
Radboudumc’s academic team continues to upgrade BabyChecker. These focus areas include:
1. Improving detection of twins: Strengthening the AI’s ability to identify multiple pregnancies.
2. Protocol optimization: Validating adjustments to the scanning protocol that could significantly improve gestational age estimation.
3. Device validation: Testing the performance of algorithms directly on the mobile app platform in field conditions.
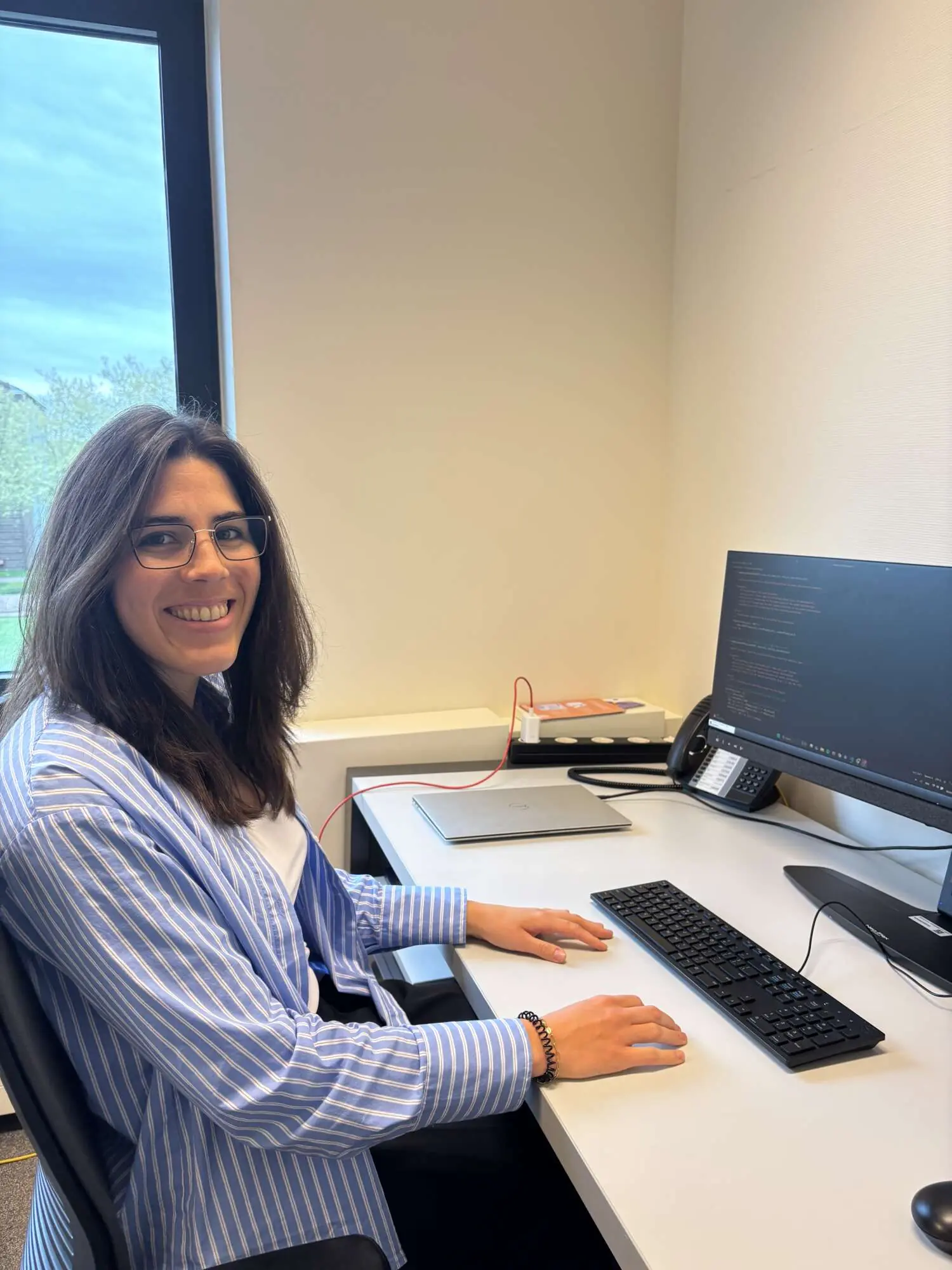
Global implementation and leadership
With Radboudumc leading academic research, we continue to work closely with local partners, healthcare providers, and public health programs to integrate BabyChecker into maternal health services.
This includes:
- Equipment delivery and setup
- Training of community health workers
- Technical support and remote assistance
- Ongoing collaboration with healthcare facilities and ministries
“When women are close to labor, having health personnel nearby can be the difference between a successful outcome or not,” said Sofia. BabyChecker enables access to vital ultrasound services, offering early identification of risks, timely referrals, and ultimately, improved pregnancy outcomes in settings where specialized care is often out of reach.
Collaboration that drives impact
The partnership between Delft Imaging and Radboudumc shows how academic research and field implementation can work hand in hand to address global health challenges. With CAD4TB and now BabyChecker, this collaboration continues to create solutions that are grounded in evidence and built for real-world use.